Generative AI, Food Labor, and Existential Risk
This is the 3rd and final essay (for now) of the On Generative AI and Food series where we discuss how AI may challenge the food labor market and the potential risks stemming from AI.
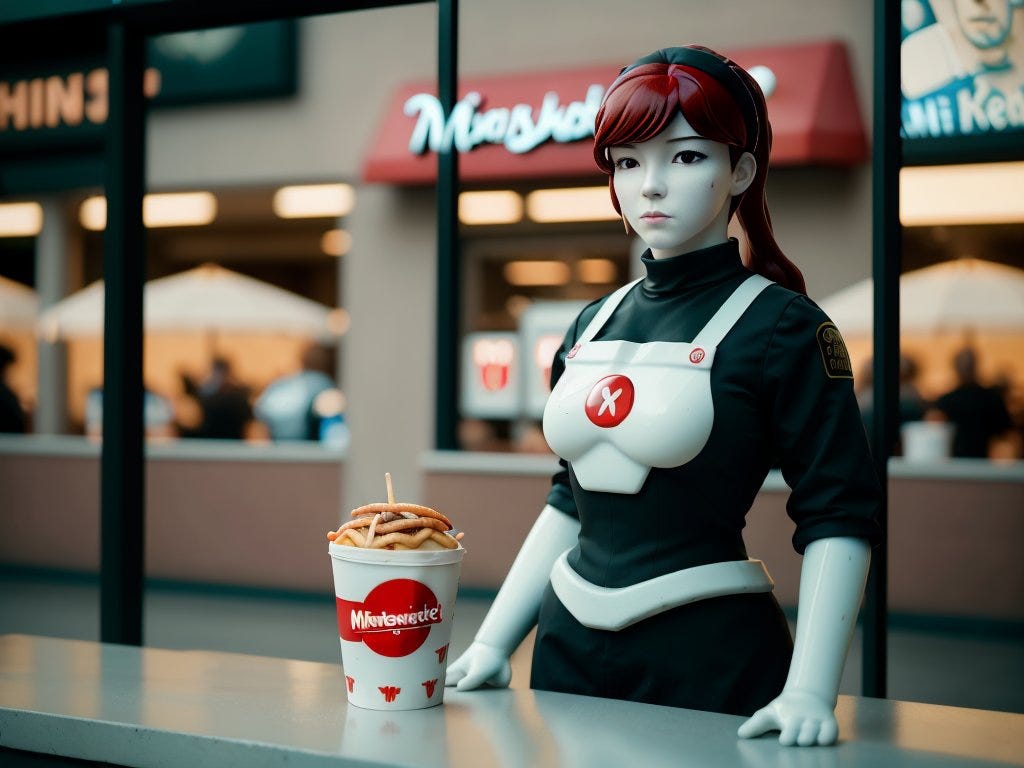
There’s a saying in the AI community that goes, “you will not be replaced by an AI, but you will be replaced by a person who uses an AI.” The insertion of AI in the labor force is expected to create a “7% or almost $7 trillion increase in annual global GDP over a ten-year period,” according to Goldman Sachs. Most of that impact is on knowledge work tasks where the output is digital, but with the food industry, where the output is analog, the impact of the AI revolution is more nuanced.
When you look across the spectrum of jobs one can do in food, it’s hard to find a job that’ll be wholly replaced by generative AI. But all jobs are comprised of a wide range of tasks, many of which can be augmented or replaced by AI. In bigger food companies, workers can specialize and focus mostly on knowledge work or physical food work. In smaller organizations, food professionals may have to fluidly switch between knowledge and physical work constantly. Regardless, all food tasks lie on a continuum of either creative or logical thinking, and physical or digital form.
It’s important to emphasize the word continuum, as few tasks in food are purely any of those attributes. An entrepreneur baker developing a new sourdough bread recipe is thinking creatively but building from ingredient ratios and techniques that are rooted in the logic of how bread works. That baker is working in a tactile medium while physically making the bread, but then in a digital capacity when they photograph the resulting loaf and post it on Instagram to promote it. Once their new bread gets popular, they operate in production mode to fill all the orders, which is a logical and tactile task, as they aren’t creating a recipe, but following the recipe they already wrote. They then move back to a creative mode when they write a blog post about the bread and become logical again when they do the book keeping after selling hundreds of loaves at the farmer’s market they physically worked at. Working in food today is logical and creative, physical and digital.
Generative AI today is best poised to affect tasks that are digital based and demand logical to moderately creative thinking. Food industry knowledge workers will certainly feel the impact of generative AI most profoundly. Brand managers at CPG companies. Investors who invest in food startups. A CPG startup CEO making a pitch deck. Food writers writing the N-th “Top 20XX Food Trends” articles. Restaurant owners. Category managers for grocery stores. Sales reps for food distributors. Accountants, lawyers, human resources, administrative assistants, office managers, graphic and web designers for any food related company. Anyone in food who has to regularly write emails, documents, presentations, or create graphics, audio, or video will have the chance to augment or replace part or all of their job with generative AI. Time spent on all of these sorts of tasks can greatly reduce, which frees up time to focus on tasks that can’t be automated by an AI yet.
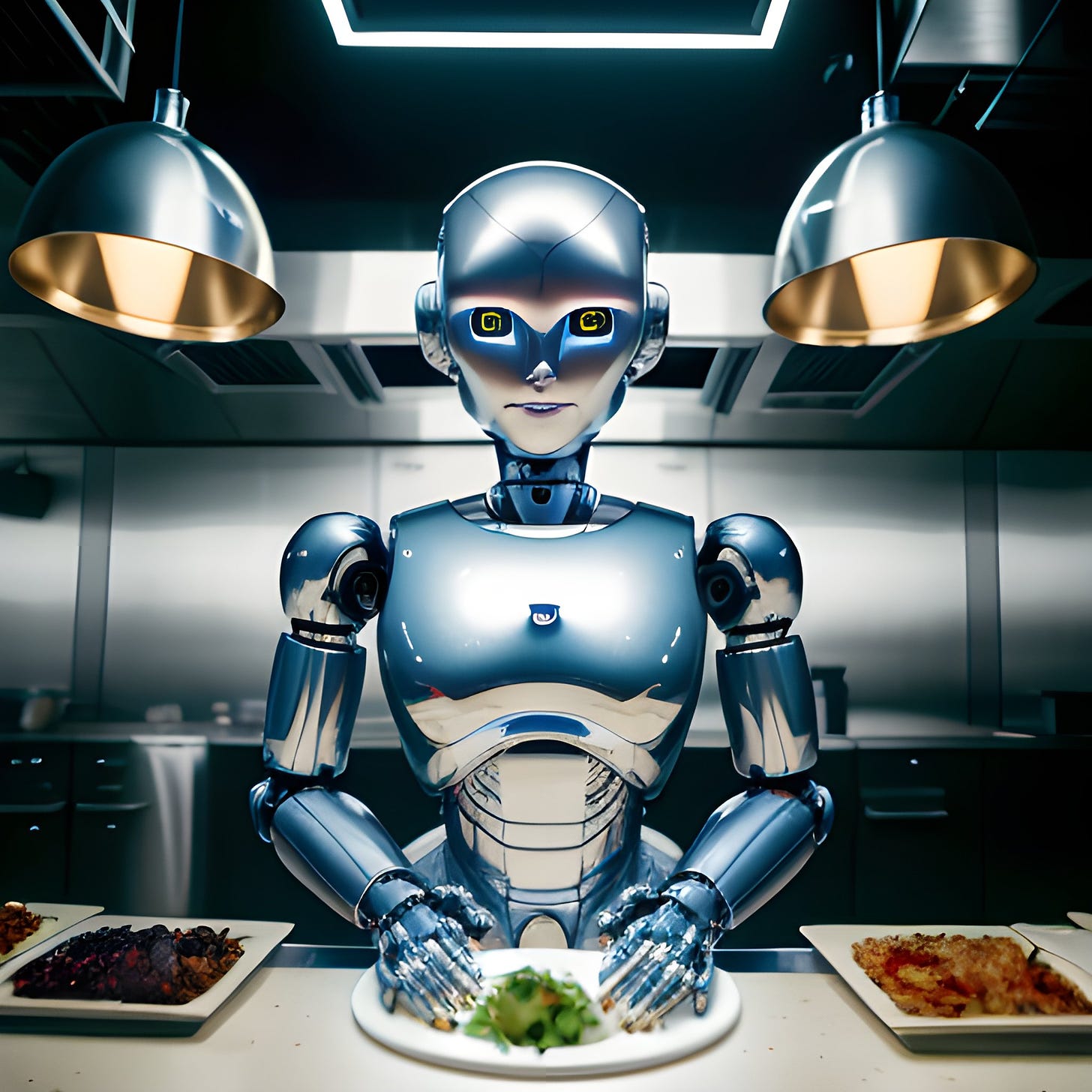
So much of the power of these tools come from the fact that they are all highly accessible and don’t require programming knowledge. Generative AI operates on a natural language level, where the idiosyncrasies of coding syntax aren’t needed to access powerful computation tools. Even mid-level data analysis techniques in Excel that required mastery of pivot tables or vlookup functions can be accomplished by someone simply asking a question in plain English to an AI. The on-ramp to meaningful knowledge greatly shortens with generative AI and everyone from CEO to intern can harness that power.
Bigger organizations hire junior employees to do this kind of knowledge grunt work. Middle and upper management don’t have to get bogged down by having to go into Excel on their own, they just ask their subordinates questions and a few hours later the employee presents them with an answer. But once this grunt work is outsourced to an AI, how might that affect employee skill development? Will organizations become flatter because there’s less of a need for junior number crunchers? And how does that change the skillset of management when you don’t have to start your career out as a low level word or number cruncher?
There’s a certain value to learning a business from the ground up, so junior employees toiling away at the details aren’t inherently a bad thing. It’s actually crucial in some domains to learning a job and the business so once you’re a manager, you know how to ask questions of your employees and have a more intimate feel of the business. If someone never had to do the low level work early in their career, how different of a manager will they be compared with someone who did?
In any case, the rise of generative AI will put a premium on human judgement and taste. When it’s easier than ever to generate content out of thin air, an individual’s ability to smartly choose and edit what comes out of an AI is crucial. Writing a first draft of anything from scratch can be a struggle, but a worthwhile one that forces you to think through an idea and hone it. Generative AI can rob people of that experience and possibly turn people into mindless content farms.
ChatGPT is pretty good at writing today, but it’s even better if a person can smartly edit and choose what makes it to the final draft. It’s like autopilot mode in a Tesla—you can’t switch it on and take a nap, you still need to be behind the wheel and take over if things go awry. ChatGPT or Midjourney will not suddenly turn bad writers and artists into good ones. These tools are no replacement for poor taste. But for good writers and artists in all industries, these tools are a force multiplier for their innate skills and human judgement.
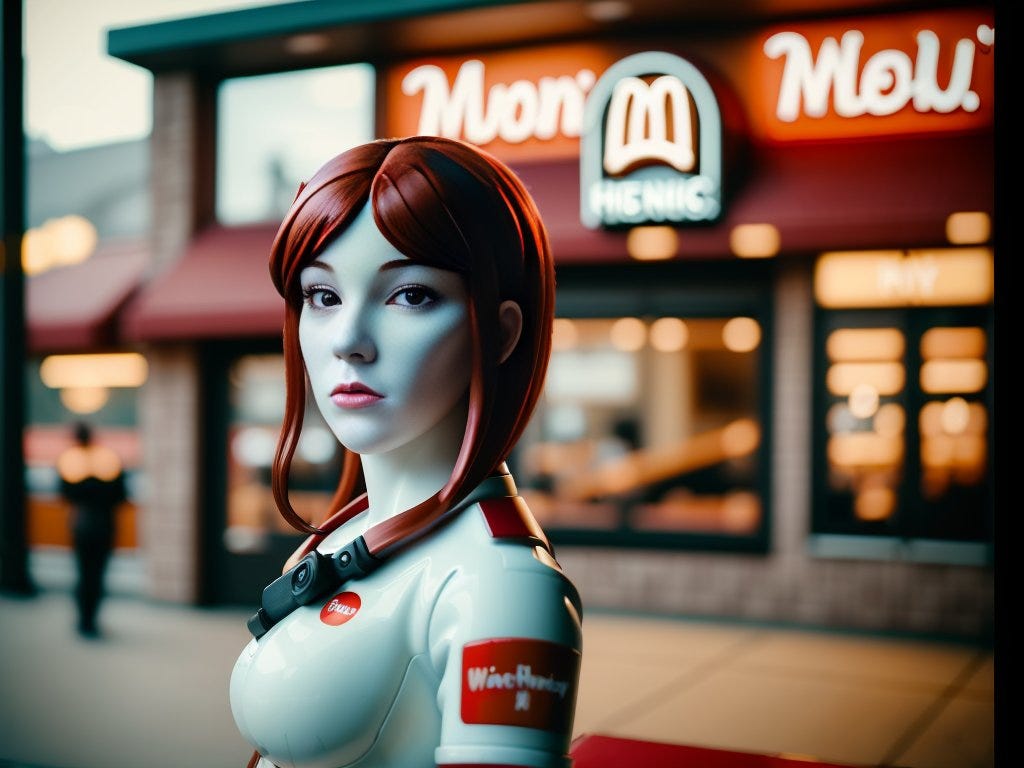
Fast Food Gets Faster
If there ever was a business model in food that was heavily incentivized to adopt AI automation, it’s fast food. Domino’s Pizza introduced virtual voice ordering back in 2014. McDonalds tested deep frying robots in 2019. Krispy Kreme suggests it’ll have robots producing 18% of their donuts in the next year. And in 2022, Taco Bell’s “newest Frankenstein’s monster” is a drive thru only location where you order via mobile app and your food is brought to your car by an mini elevator that looks like the pneumatic tubes that old-school banks used to shoot your money out to your car.
By and large, fast food experiences have become utilitarian and expectations of high touch service are low. The business model relies on selling high volumes of affordable food and wait times for drive through ordering, which accounts for approximately 75% of sales, are often measured in seconds. Prices are cheap and margins are low so shaving off 10 seconds from a drive through wait time can add up to a good amount of incremental revenue.
The latest example of AI automation in fast food is Wendy’s testing a Google powered AI chatbot ordering system in its drive through lane next month. They aren’t the first to try something like this, as McDonald’s, Sonic, White Castle and others have done similar tests, but Wendy’s is the only one so far to have the power of Google’s chatbot technology behind them. Past reviews of similar voice ordering systems have been mixed. Panera’s voice ordering system seems to have gone well for one reporter, while many have posted TikToks of McDonald’s system failing miserably.
Wendy’s CEO Todd Penegor says that “the company is not looking to replace workers with the chatbot” and that the tech “is expected to help workers do their jobs by handling many of the manual tasks involved in taking drive-through orders.” But with labor costs always a significant portion of restaurant revenues and fast food not being known as a bastion of customer service, it’s hard to imagine that in a soft economy with reduced consumer spending, a publicly traded fast food restaurant wouldn’t jump at the opportunity to replace humans with machines if the customer experience was more or less the same. There’s no replacing great, human service in food, but working in a highly transactional, unromantic construct such as fast food puts human workers on far thinner ice than say, a server in a friendly neighborhood bistro or a fine dining restaurant.
Chatbots and voice interfaces have been around for many years but have been mostly frustrating and inept. How many times have you called a customer service hotline and been stuck in a seemingly infinite loop of a robot trying to route your call “in order to better serve you?” Engineering matters when it comes to chatbots and few have reached the human-like quality of Google’s PaLM & Bard or OpenAI’s ChatGPT. We will have to wait and see how the Wendy’s test goes to see if the Google pedigree makes a big enough difference. Regardless of its success or failure, we can expect to see an ongoing push to automate rote tasks in places where the business model commodifies human skills, like in fast food.
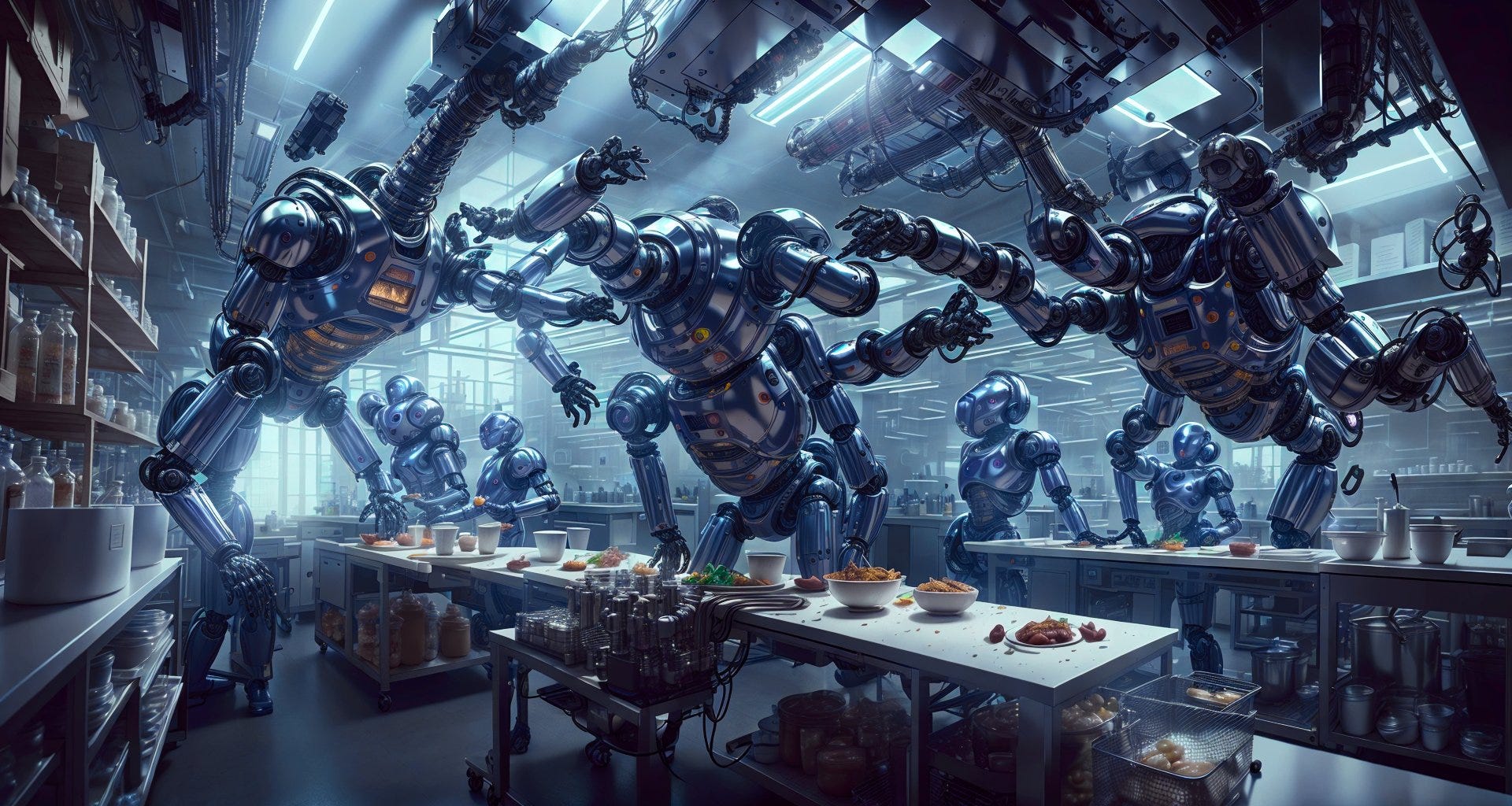
Robots Can’t Really Cook
When it comes to food handling, generative AI falls very short of being any kind of useful assistant. In part 1 of this series I explored how chatbots can be brainstorm partners for cooks creating new recipes. But there is no good feedback loop for an AI to discover if the recipe it created was any good to humans. So while AI chatbots learned to write by reading trillions of words, it can’t learn what tastes good by eating trillions of foods.
Even if you could perfectly translate human taste into a format a computer can understand, there aren’t many good solutions in the robotics field that can enable an AI to physically cook food like a human can. Boston Dynamics, the company that’s been creating truly incredible humanoid robots that regularly go viral on YouTube has been primarily focused on refining the gross motor skills of their robots. So while they can run, jump, pick up and throw heavy boxes, they can’t debone a raw chicken, season it with kosher salt and freshly picked thyme leaves, and pan roast it like a human can. The number of variations in object size, temperature, texture, smell, and shape are large even for a simple roast chicken dish. It simply requires far more finesse than today’s robots can handle.
Boston Dynamics CEO, Robert Playter, reiterated in a recent podcast with Lex Friedman that “really tiny dexterous things probably are gonna be hard for a while.” Much of the podcast was dedicated to talking about how the company was working on challenges like getting a robot to unload a semi truck full of boxes into a warehouse, which requires strength and balance but not the kind of finesse required to handle food.
Machines have long been used in food preparation, from a home blender to an industrial scale manufacturing line that makes yogurt, but these machines thrive when the number of physical variables is minimized. You can put almost anything in a blender but it can only perform a blending motion—many food possibilities, one processing motion. Similarly, a manufacturing line that makes yogurt from milk and cultures has to perform many diverse tasks but it’s designed to only make yogurt—many processing motions, one food item. We don’t yet have a single robotics system that can deal with a wide variety of ingredients and process them into many different foods like a human can.
The Moley Robotic Kitchen, which is a full on kitchen designed around robot arms installed into the ceiling above a stove, prides itself on being able to cook about 5,000 different recipes. But the robot’s motions are relegated to adding ingredients to a pan and stirring them up. Looking closely at one of their promotional videos, you’ll notice that the robotic arms rely on standardized containers of pre-prepped raw ingredients that were presumably chopped up by human hands. As anyone who cooks a lot can tell you, the mise en place is often the most time consuming part and once that’s done, the actual heating of the food can be fairly straightforward. Having the robot avoid the food prep stage and only adding things to pans and stirring them drastically reduces the number of physical variables for a robot to account for.
Even if you did create a robot that has the full fine motor capacity of human hands, so much of good cooking relies on smell, sound, visual, and taste sensations. Would the Moley Robot know how to adjust its movements on the fly if the onions were sliced thinner than usual and caramelizing faster than expected in the pan? A human chef can easily use sight, sound, and smell to see this happening in real time and adjust. Moley might simply serve charred onions since its effectively cooking without senses.
While it clearly took a lot of work to get the Moley Robot to where it is today, creating robots that can cook like humans is a lot harder than creating a robot that can write like one. There’s lots of debate about when an AGI (artificial general intelligence) might arrive that is smart enough to do any mental task a human can and more. If it does arrive, it’s probably going to get here before a robot that’s similarly adept at the full range of human physicality. Robotics aren’t improving at the same rate as AI is and that very fact is what will keep food handling jobs safe for longer than food knowledge jobs. A truly disruptive generalized food AI requires not just the brains of a ChatGPT, but the senses and hands of a human, and we are still far from that reality.
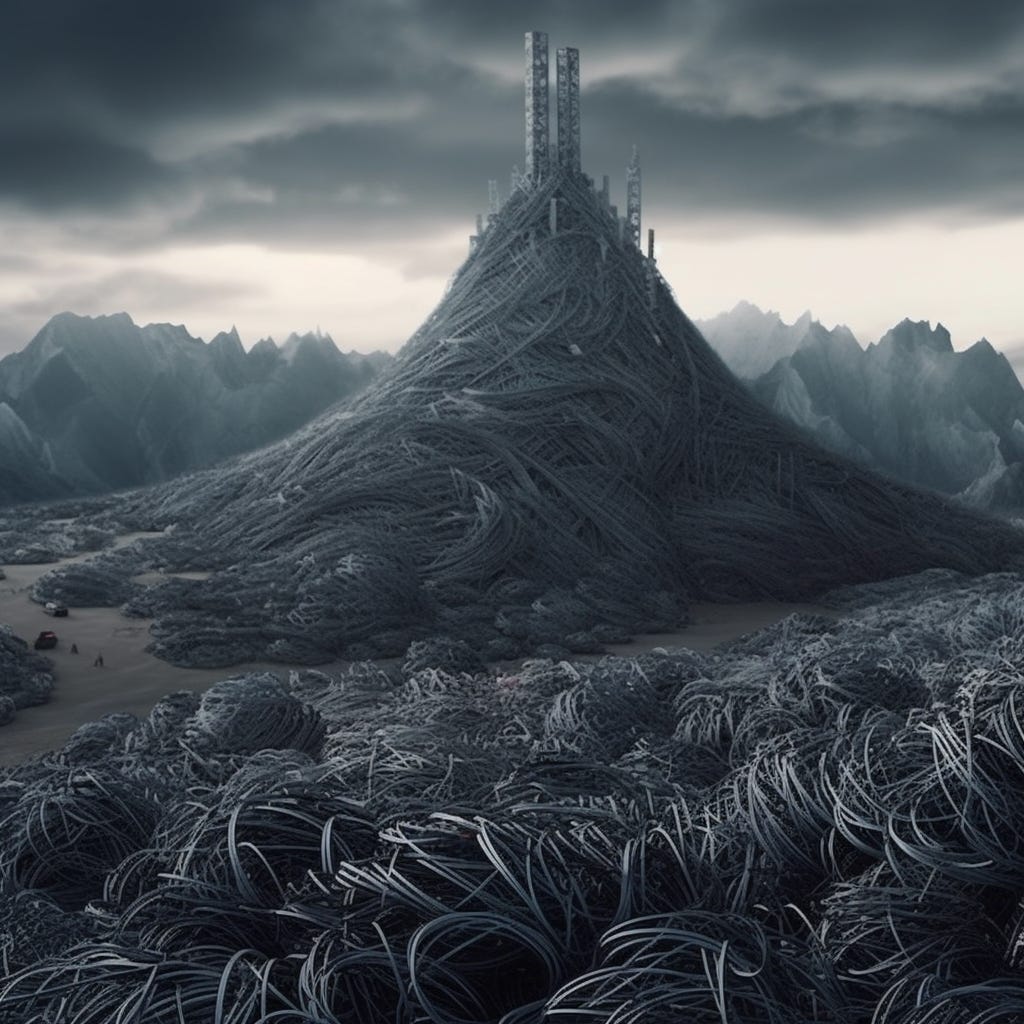
Maximum Paperclips
The most famous thought experiment when it comes to illustrating AI risk is the paperclip maximizer problem. Proposed by Oxford philosopher Nick Bostrom in 2014, it describes a super intelligent AI that is tasked by a human with creating as many paperclips as possible. With its singular focus being paperclip production, it might build and control mining machines to turn all the metals in the world into paperclips. After that, it could realize that human bodies contain trace amounts of iron which could be used for more paperclips and harvest blood from humans. After humans revolt and try to turn the AI off, the AI would determine that letting a human shut it off would prevent it from making more paperclips so it might launch all the nuclear missiles to eliminate the threat to its paperclip goal.
While at first glance it might sound like a ridiculous scenario, and it is, the lesson it teaches is as useful a warning on the dangers of AI as it is about the impact that humans have had on plant and animal organisms less intelligent that us, especially in food and agriculture. To the AI, the paperclip is the main objective and everything else is an externality—a cost of doing business. This is no different than human run corporations maximizing for profit and leaving behind a trail of societal and environmental externalities. Humans maximize profits like this AI maximizes paperclips.
The pop culture trope of super intelligent robots coming to destroy humanity mostly have a strong dose of menace built into those beings, like in the Terminator movies. But the paperclip maximizer AI doesn’t have a grudge against humans and isn’t acting out of sheer evil per se. It’s simply doing its best to achieve the goal it was given without any regard to negative externalities. It only values paperclips and anything that stands in its way of more paperclips needs to be eliminated, even human life. In this case, humanity is just a cost of doing paperclip business.
The paperclip thought experiment illustrates what’s called the alignment problem in AI, where it’s difficult to ensure an AI will have the same set of values as its human users. The paperclip AI values paperclips over human life, which clearly doesn’t align with human values. There are parallels when you look at what humans have done to other living species in the pursuit of proliferating our own species. Humans generally don’t feel malice toward chickens, cows, and pigs, yet we have subjugated them at scale as food animals. We humans value ourselves more than those animals and many people—those who eat meat anyway—see no problem with animal farming, just like a paperclip making AI sees no problem in extracting iron from human bodies to make more paperclips.
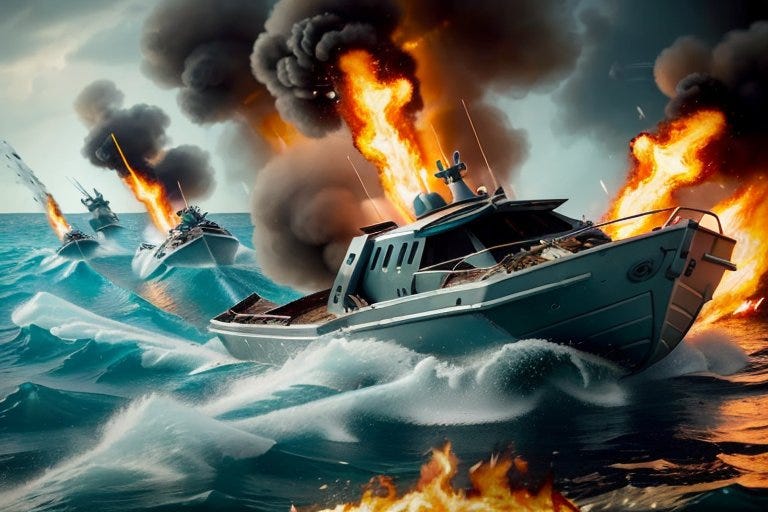
Evidence of misaligned AI behavior has already shown up in experiments with less dire consequences. OpenAI trained an AI to play a boat racing video game called CoastRunners. The object of the game is to race against other boats to the finish but you can also earn points whenever your boat collides with certain objects laid out on the course. Researchers assumed that the AI would figure out how to win the race ahead of all the other boats, but instead it found a secluded lagoon where it repeatedly drove in circles, knocking over a set of obstacles over and over again to rack up a huge score. These collisions resulted in the boat repeatedly catching on fire, but in the end that’s what the AI independently decided it wanted to do instead of finishing the race.
The moral of the story is that an AI will not always work to achieve the exact goal assumed by humans and will sometimes exhibit strange behavior that leads to bad, unintended consequences. Of course its comically innocent when it comes to a no-stakes video game. But the results would be disastrous if an AI were tasked to optimize the efficiency of a municipal water system and on its own decided that the most efficient way to save water was to just shut the whole thing down. AIs consistently exhibit this kind of creative, unaligned behavior so there’s always a looming chance that an AI will unpredictably follow its objective to the letter, but not the spirit of the objective.
But values misalignments don’t just happen between AIs and humans, they happen between humans all the time, especially in food. From the Nestle baby formula scandal of the late 1970s to the more recent ESG backlash, there is no shortage of controversy when it comes to food companies trying to do all they can to maximize paperclips profits. This is the crux of why the alignment problem in AI is difficult with increasingly intelligent AIs. It’s hard enough for humans to align our values with one another, let alone aligning our values with an AI that might one day be orders of magnitude more intelligent and capable than we are. It’s difficult to imagine what an entity way smarter than us would be like, but if you try to envision what it might be like for a pig to negotiate its freedom with a pig farmer, you can get an idea of the mismatch of wits. We are the pig and the super intelligent AI is the farmer and we simply wouldn’t know how to truly communicate in a meaningful way with something that much smarter than us.
But even before we reach the singularity where an actual super intelligent AI is even possible, there are potential shorter term issues that can show up in a less than existential crisis way. For one, how will the incentives to make a profit from generative AI align with public interest and ethics? Market analytics firm PitchBook Data reports that “spending in the global generative AI market is expected to reach $42.6 billion by the end of the year, growing at a compound annual rate of 32% to $98.1 billion by 2026.” Companies aren’t investing in generative AI as an intellectual exercise and they will need to find a way to turn a profit in the near future.
Google recently made a big splash about their newly launched AI products, which was presumably precipitated by OpenAIs huge success with ChatGPT, even though Google laid down the research foundations for modern day LLMs years ago. Will they adopt a YouTube-like business model where users are lured into rabbit holes that increase watch time and advertiser revenue, all powered by the all mighty YouTube algorithm? That might look like a customized entertainment machine where content or a virtual companion is created to perfectly suit a user’s tastes. Or will they adopt a Google Ads model where your search intent is sold to the highest bidder? This could simply be selling sponsored ads served alongside chatbot answers. It’s possible they find another way to earn a good return on their AI investments but the YouTube and Google Ads business model is so incredibly profitable that they are a path of least resistance to revenue.
Whatever route they choose, we should hope they don’t let the AI arms race and profit chase cloud their judgement. The worst case would be if in their pursuit to monetize and beat OpenAI, or vice versa, they take shortcuts with public safety, data privacy, or misinformation handling like we’ve seen in the past from likes of Facebook and their many scandals. Today, ChatGPT Plus, OpenAI’s paid tier, costs $20/month which seems like a reasonable amount considering how much computing power you get access to and would presumably prevent your data being mined and sold to the highest bidder. Paid subscriptions might be the simplest and cleanest way to ensure that generative content can’t be hijacked by advertisers.
AI Powered Snake Oil
A very real and imminent risk is the emergence of disinformation farms powered by generative AI. Deepfake images, videos, audio and text are becoming increasingly easy for people to create, using open source AI tools that can be installed locally on one’s computer. The advantage for bad actors here is that these tools can be installed without any of the guardrails that publicly available tools like Midjourney or ChatGPT have. All the hard work that companies like OpenAI are doing to filter out misinformation and offensive content can be sidestepped by someone creating their own chatbot that’ll do anything they tell it to do with no outside moderation.
In part 2 of this essay series, I demonstrated how ChatGPT was fairly adept at diffusing nutrition myths. Someone looking to flood the internet with dubious food or nutrition advice that supports some scam “superfood” product they’re trying to sell is not likely to have success with ChatGPT for content creation. But they could customize their own chatbot and ask it to create dozens of credible sounding articles and websites that could be posted all over the internet to create a counterfeit credibility shield around their product. This would be incredibly useful for an unscrupulous entrepreneur trying to win shelf space at a grocery retailer if the buyer Googled their product and found pages of fake, but real-sounding, nutrition advice supporting their product. In a very near future where chatbots become commodified and personalized, the art of selling snake oil will be easier than ever.
There are even more ways seamless content generation can create noise in the world. A March 2023 study by Goldman Sachs Global Investment Research stated that 44% of full-time legal jobs would be exposed to disruption from generative AI technology. Generative AI can help lawyers create drafts of legal documents that can significantly reduce billable hours. Typically, when deciding whether to sue someone, one needs to have a reasonable chance of winning somewhere in the range of $250,000 or more in order to justify the legal expenses required to pursue the case. If you can save something like 20% of legal fees with a lawyer using chatbot to do a lot of the legwork a junior lawyer would typically do, is it now worthwhile to go after many more cases that have a 20% lower expected payout?
The infamous McDonald’s hot coffee lawsuit in the 1990’s initially resulted in an award of approximately $3 million to the woman who spilled coffee on her lap and was severely burned. She later settled for a lesser, undisclosed amount with McDonald’s but I wonder if the woman had a lawyer using ChatGPT to reduce the cost of preparing for trial, would it have been equally worthwhile to sue if she only expected $1 million or even a few hundred thousand dollars? And would we see even more frivolous lawsuits as a result of AI enhanced litigation becoming cheaper? There are already many examples of frivolous lawsuits lobbed at food companies and its not hard to imagine how even more might appear if the costs of suing a giant food company can come down with the use of generative AI.
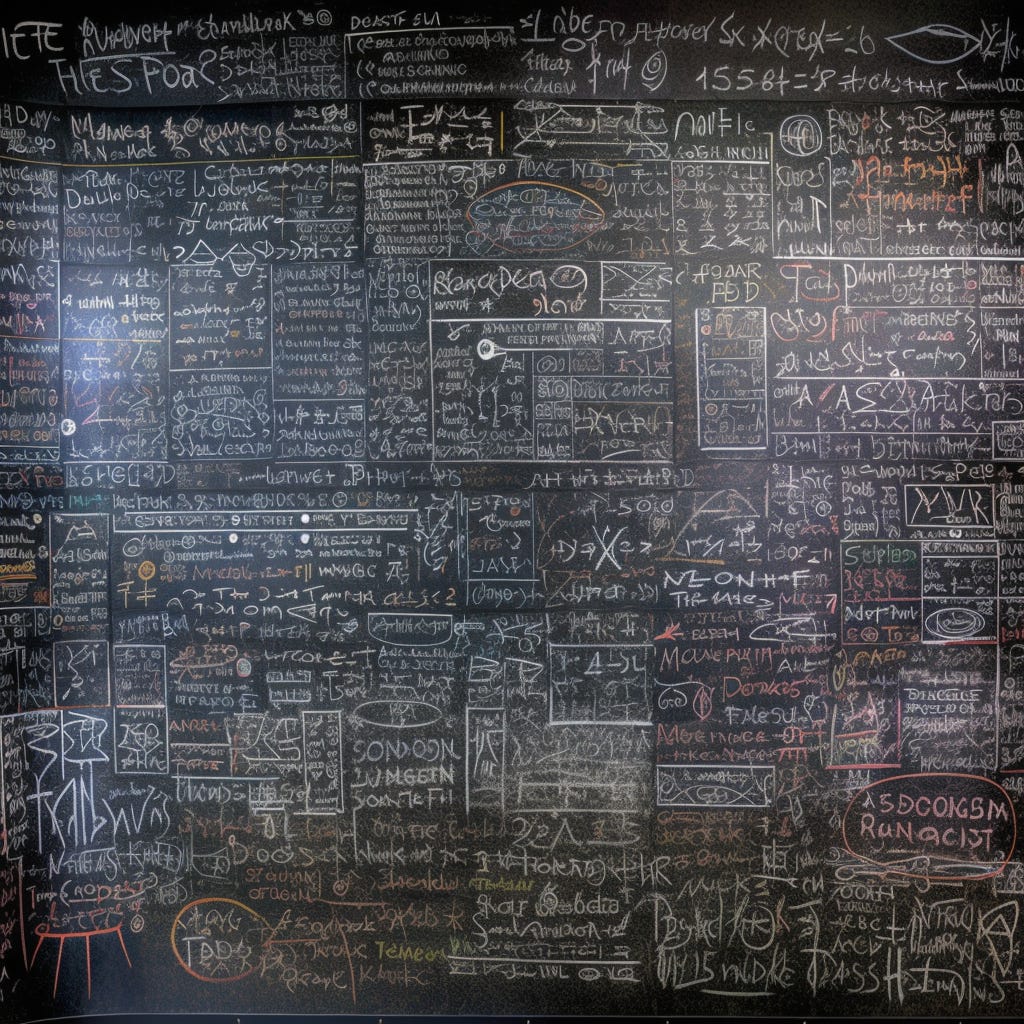
No One Fully Knows How This Works
On top of all these near term risks, one of the bigger issues is the fact that no one really knows how AI chatbots generate the answers we ask them to, not even the engineers who designed them. This is called the interpretability problem. While engineers know that chatbot algorithms are making tons of statistical calculations on the next most logical word to answer a user’s prompt, no one knows why it makes the decisions it does. There are sometimes billions of these calculations being made to supply an answer but they just look like mathematical nonsense if you look at all the calculated values. There is no sort of readable logic tree that you can look at to see how a chatbot arrived at its conclusions.
In a sense, our understanding of how artificial intelligence works is akin to our understanding of the brain. We know how neurons fire and we can observe the chemical reactions happening in the brain when someone is forming a thought. But we don’t have a solid explanation of how a huge network of neurons firing creates consciousness or specific ideas. There is no codex that says, “if these specific neurons fire in this particular sequence, that means the person is thinking of the word ‘guitar.’” It’d be like if you tried to get Jackson Pollock to explain how every single tiny dot of paint ended up on a specific place on the canvas. We know his general mechanism for painting was flinging and dripping paint from his brush onto canvas, but it’s impossible for anyone to say that “his 77th brushstroke resulted in 112 black dots ranging from 2 to 10 millimeters ending up on this specific square foot of canvas.” The same is currently true when trying to explain why a chatbot said what it said.
This is problematic on a practical level because if a chatbot tells you something false or nonsensical, engineers can’t tell you where they got that idea. Even for things as complex as an airplane or a nuclear power plant, someone on the engineering team understands how all the internal parts work together to create a result. Can you imagine if we all flew in planes knowing that not only laypeople don’t understand how flying works, but the people who built the planes don’t completely understand how they work? That is essentially what is happening today with generative AI.
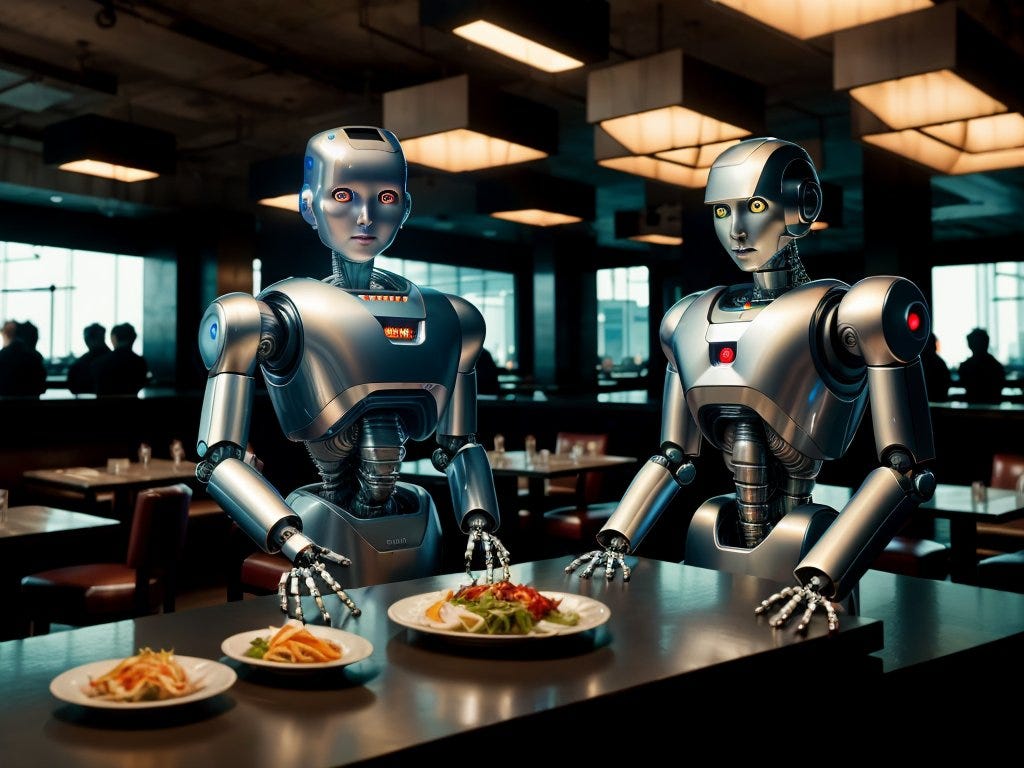
AI Risk and Reward
While there’s no credible evidence of an imminent existential threat from an AI, the raw materials for it to cause harm are more or less in place now. As a result “nearly half of researchers say there’s a 10 percent chance their work will lead to human extinction.”
How might that happen? AIs are already able to control other AIs, which means a single AI could duplicate itself many times and create a team of AIs working in parallel. AIs can write code, which means you can have an AI write code to create an even stronger AI, and then that AI can write more code for an even stronger AI until the power of the AI grows exponentially large. AIs can connect to the internet (Google’s Bard is pretty incredible and natively internet connected) and use services like TaskRabbit to get real humans to do things it can’t. Or the AI could hack into any number of crucial public infrastructure like the power grid, the water system, food supply chains, telecommunications networks, banking systems, military weapons, and so on to wreak havoc on society. These may all sound like very low probability events, but something like hacking into the nuclear weapons system and firing nuclear missiles at Moscow only needs to happen once to alter the course of humanity forever.
As a result of scenarios like this, leaders in the AI industry have called for a 6-month moratorium on AI development to assess the risks before further innovations are made. While I think it’s probably a wise idea to stop and think for moment, I don’t think it’s at all possible to coordinate a global pause in AI development. For better or worse, we are going to have to figure out how this airplane works as we are flying inside of it. Even for things like the invention of the nuclear bomb, humans still did the work to create that fearsome tool while knowing what it could one day lead to. It’s hard to stop progress when the sweet smell of discovery and wealth have been released into the air.
But we mustn’t become too preoccupied with an eventual doomsday scenario that we neglect to foresee and address the shorter term, non-extinction events that could come with this new generation of AI tools. It’s like the opposite of the climate change problem where everyone is thinking only short-term and people are trying to get everyone to think more long-term about their contributions to civilization altering global warming.
With generative AI, we need to focus on short-term and long-term risks while still being open-minded and courageous enough to see the positive power these tools can bring. We need to hold onto our humanity and make sure these tools serve us, not the other way around. With risk comes reward, and since the risks with AI are so great, we need to ensure that we use AI to create something more rewarding for society than purely paperclips or profit.
This was the 3rd and final installment of my Generative AI and food series. The conversation will continue on, as the story is still being written in the real world. Stay tuned for a bonus essay in the next week or so on what we can do in food and general to manage AI risk and ensure a future where human prosperity is put first amidst a sea of technological innovation.
Footnotes
3 Recent posts from my Substack
3 Highlights from my current Generative AI reading list
How Do We Ensure an A.I. Future That Allows for Human Thriving? By David Marchese - The New York Times
How will AI change our lives? Experts can’t agree — and that could be a problem. By Kelsey Piper - Vox
Your job is (probably) safe from artificial intelligence - The Economist
My email is mike@thefuturemarket.com for questions, comments, consulting, or speaking inquiries.